The Importance of Sanity Checks
Data so outrageous that you should know at first glance it's wrong
It’s been a little while since my last post. Between a winter vacation and a project I’ve been working on (I hope to share more on this soon!), I’ve gotten a bit behind. Plus, it seems like every time I have something I want to write about, another new thing comes along before I get a chance to sit down and actually write it up. But there’s been a common thread that runs through many of these recent topics, so I thought I would address a few things at once.
A lot of people ask me how I find the Covid data errors that I point out from the CDC, the media, and on Twitter, or how I know they’re wrong. And it basically comes down to asking the question, “Does this sound plausible?” In order to answer that though, you must be familiar with the data. I can’t fact check tweets or articles about baseball, for example, because I don’t have the requisite knowledge base. I wouldn’t know if a certain statistic sounded right or not. But over the past three years, I have gotten very familiar with a lot of Covid data, so many errors jump out at me because they simply don’t pass the sniff test.
Long Covid
This week, Laurie Garrett tweeted that “84.2% of #COVID19 survivors in a Swedish cohort reported persistent symptoms affecting daily life 2 years after hospital release,” and I immediately knew that wasn’t right. Unfortunately, too many science journalists and supposed Covid experts either aren’t capable of sanity checking like this, or they aren’t interested in doing so. In this case, the 84.2% number came from a survey of a small group of people who were hospitalized with Covid in Spring 2020 AND who reported Long Covid symptoms 4 months later. Many people replied to Laurie to point out this detail, but she ignored all the responses about her misleading tweet. (I will give credit to CIDRAP, who actually did acknowledge my feedback and update their article today.)

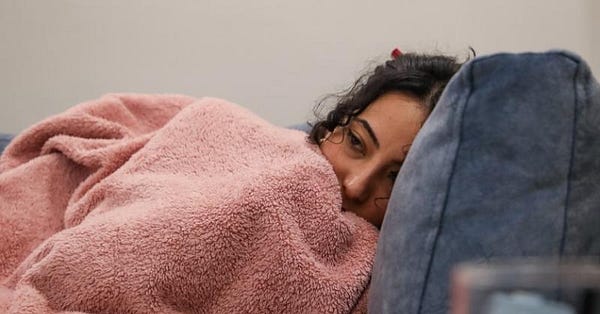
Handy tip for evaluating Long Covid estimates:
If you see a statistic on Long Covid prevalence anywhere near 80%, assume there’s an error.
Quickly sanity checking other claims of up to 80% having Long Covid is also how I was able to find errors in these flawed Long Covid papers. Unfortunately, the authors of both papers have ignored my messages. Almost everyone has had Covid at this point, and it’s blatantly obvious that 80% of the world isn’t suffering from Long Covid, yet multiple articles and studies have cited that absurd percentage. Meanwhile, the CDC Household Pulse survey finds ~6% of U.S. adults are currently experiencing Long Covid. Estimates of Long Covid prevalence should be much closer to 6% than to 80%. Even among hospitalized patients, who make up a small fraction of Covid patients, it’s still nowhere near 80% who experience Long Covid. And studies based on Covid cases identified via electronic health records (EHR) are also biased, because more severe cases or people with more underlying medical issues are the ones who test at doctor’s offices and hospitals.
I even suspected that this headline was wrong when I saw it on Twitter: “16% of all North Carolinians have long COVID”. Turns out, it was based on the CDC Household Pulse survey, but the survey found 16% of people in North Carolina have ever experienced Long Covid. That would include people who took a little while to get your sense of smell back in 2020. Despite attempts to contact the journalist and the paper, they did not update their inaccurate headline.
Mask Studies
Like Long Covid, many mask studies done during the pandemic have dramatic results that don’t pass a basic sanity check. Even if you believe strongly that masks are capable of blocking viruses, we’ve seen over and over again that mask mandates have not prevented huge waves, and we’ve also that many locations with different mask usage have had nearly identical curves. Ian Miller made a name for himself sharing mask graphs on Twitter illustrating this observational data.
So when mask studies show results significantly different than what we’ve observed all over the world the past three years, treat them with suspicion. For example, the infamous CDC MMWR on masking that showed 56% lower odds of testing positive when wearing a CLOTH mask. Many people uncritically share that study still today, as if it’s undisputable evidence that masks work. Wise observers laughed at the huge effect size, and then noticed that it was based on a survey with low participation rates, and that the 56% result for cloth masks was not statistically significant.
A recent example came from a school masking study in San Diego, which was presented at the CROI conference. Based on school-level wastewater monitoring and observations of student masking outside after school, they claimed that a 10% increase in masking resulted in an almost 50% decrease in the odds of finding Covid in the school’s wastewater. These numbers are highly dubious and should set off alarm bells for anyone who has followed masking studies with an open mind. Even the author said she was surprised by these findings. Not surprised enough to find out how she could have gotten such implausible results though! From her Twitter feed, it’s clear she’s a hardcore mask devotee, so her incurious nature about these study results isn’t shocking. There is still no paper written on this study to reference, but from her own slides, it’s clear that there’s more that meets the eye with the results.
The team observed and monitored wastewater at 10 schools on 6 separate weeks. Of those 60 observation events, only 9 detected ANY Covid in wastewater (likely because many students and teachers do not poop at school). As shown in the slide above, much of the mask wearing was in March, when a mask mandate was in place for San Diego United School District, and when cases were approaching record lows. That mask mandate ended April 4, when the students returned from Spring Break. Two signals were detected in school wastewater the week after Spring Break (cases contracted over break?), and 5 of the 9 signals were detected in May, when community case rates were climbing and much higher than they were earlier in the study period.
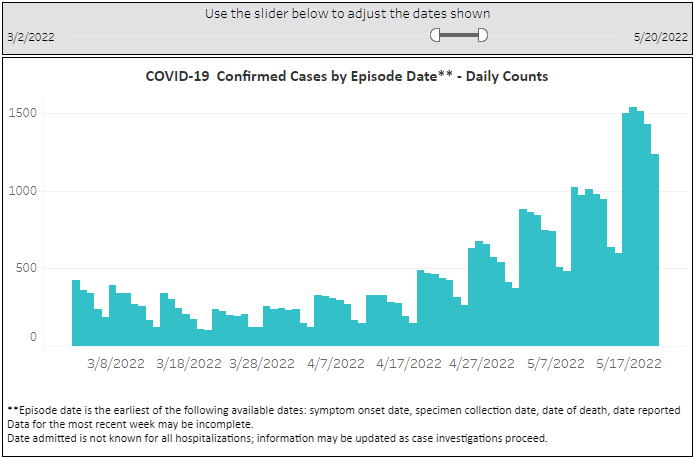
Timing was actually one of my initial suspicions when I saw these findings, because that is a common issue with many observational mask studies. If you choose the right window and implement a mask mandate as cases are peaking or drop a mask mandate just before cases rise, you can easily show a correlation between the masks and the cases. But of course, correlation doesn’t equal causation. Studies often report that they’ve adjusted for confounding factors like case rates, but it’s impossible to fully resolve all of the confounding variables.
I’ll write more about this study if a paper on it ever comes out, but of course, much of the damage from it has been done. It was spread widely by journalists who uncritically accepted the findings without question, even though it’s an implausibly high effect size for a small amount of cloth mask wearing by young children. For example, this Healio article about the study was promptly sent via email newsletters to members of the American Academy of Pediatrics (AAP)1 and the Infectious Disease Society of America (IDSA)2 and shared widely on Twitter. While some people called out issues with this study, too many didn’t bother to see if the findings seemed even remotely realistic. If it seems like doctors often speak with one voice, perhaps it’s because they’re all being fed these type of poorly evidenced stories from the media on a regular basis.

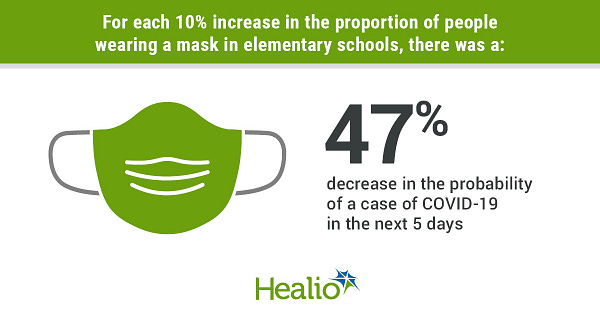
Pediatric Covid Data
A third area where data is often so obviously wrong that it doesn’t pass even the most basic sanity check is with hospitalization and mortality data for young people. There have been many cases of this throughout the pandemic, both from the CDC and the media. For several months, one CDC webpage showed a graph that indicated 4% of Covid deaths were in children. Anyone should have realized that was obviously false! The calculation from their own numbers when that graph was first posted showed it was supposed to have been 0.04% - it was literally 100 times too high! That leads me to believe the people who updated and approved that webpage either didn’t have the underlying knowledge to recognize that 4% was an impossible figure, or they simply didn’t bother to check if the data made any sense (like noticing that the percentages add up to 104%).
More recently, a CDC ACIP slide cited 1489 deaths COVID-19 deaths in children 6 months to 17 years. However, anyone familiar with pediatric mortality data from the CDC’s NCHS (which was cited on the slide as the data source) knows that total pediatric mortality has been between 1400-1500 for a couple of months, and the graph clearly showed that ~300 deaths were in children under 6 months, so it was obvious they used the total number of pediatric deaths, without removing young infants as the label on the slide indicated. These numbers are something I’d expect a senior CDC official, and head of the ACIP Covid vaccine working group, to be well aware of, as well as all the people that surely reviewed these slides before the meeting.
In addition, CDC Director Walensky recently testified to Congress that 2000 children have died from Covid-19, shortly after Medhi Hasan tweeted that same statistic. However that number is based on the flawed Data Tracker (as I addressed in my early Substack). Compared to actual data from pediatric death certificates via NCHS / CDC WONDER, the Data Tracker has always overreported pediatric deaths. This is yet another example where awareness of the actual data makes these errors easy to identify that anyone who makes these “mistakes” is either ignorant of the accurate data or they are being purposely misleading. This is troubling for a member of the media, but it’s especially concerning when it’s the CDC Director herself.
There have been many more instances in the media where pediatric Covid data was grossly overstated, such as when Apoorva Mandavilli reported in the New York Times that there had been 900,000 pediatric hospitalizations in U.S. (corrected to 63,000), or when she claimed 4,000 children ages ages 5-11 had died of MIS-C, when the total number of deaths from MIS-C was 68 (for ages 0-20). In August 2021, the Texas Tribune reported that over 5,800 Texas children had been hospitalized in a week due to Covid (actual number ~280). (Interestingly, the same erroneous statistic was reported in articles in the Houston Chronicle and The Daily Beast that same day.)
And let’s not forget when Supreme Court Justice Sonia Sotomayor famously claimed during a case about vaccine mandates that over 100,000 children were hospitalized in serious condition with Covid-19. When asked about it later, CDC Director Walensky declined to call out this misinformation!
In Summary…
I’ve covered three main areas where scientists and journalists routinely get Covid data factually wrong — Long Covid, mask studies, and pediatric Covid metrics — where the claims just don’t pass the sniff test. In many cases the numbers are so incredulous that random Twitter users can identify the errors easily. People often send me tweets or articles with a request to fact check the data. They know that I have the actual data at my fingertips to set the record straight and often to contact the authors and publications to make corrections.
We should expect more from the CDC, from academics, from science journalists, and others. But sadly, innumeracy is common, and many people have very skewed perspectives on Covid so that they blindly accept whatever big numbers they see. Many times, numbers have been off by orders of magnitude because people either lack the expertise to question the data, or they lack the curiosity to investigate further.